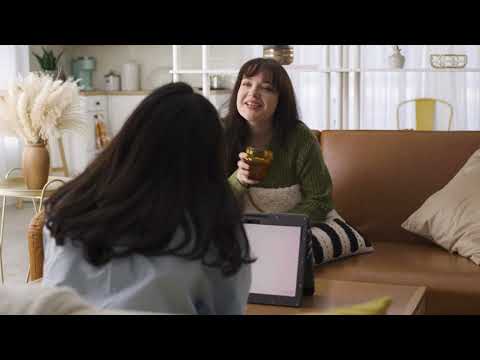
Can you imagine using a keyboard where it took a key press two seconds to show up on screen? That’s the typical latency for most voice interfaces, so it’s no wonder they’ve failed to catch on for most people. Today we’re open sourcing Moonshine, a new speech to text model that returns results faster and more efficiently than the current state of the art, OpenAI’s Whisper, while matching or exceeding its accuracy. The paper has the full details, but the key improvements are an architecture that offers an overall 1.7x speed boost compared to Whisper, and a flexibly-sized input window. This variable length input is very important, since Whisper always works with 30 second chunks of audio, so even if you only have a few seconds of speech you have to zero-pad the input and process much more data than you need. These two improvements mean we’re five times faster than Whisper on ten second audio clips!
To understand what that means in practice, you can check out our Torre translator. The speed of Moonshine means we can offer almost instant translations as people are talking, making for a conversation that’s much more natural than existing solutions.
Even better, the low resource demands of Moonshine allow us to run everything locally on the device, without any network connection, safeguarding privacy and letting us run anywhere in the world, instantly.
We founded Useful to help machines understand us better, and we’re proud to share this new step forward in speech to text, since voice interfaces are a vital part of that mission. Moonshine doesn’t just help us with products like Torre, its unique design makes it possible to fit full automatic speech recognition on true embedded hardware. We’ve found the biggest obstacle to running ASR on microcontrollers and DSPs hasn’t been the processing power, since accelerators help with that, but RAM limits. Even the smallest Whisper model requires at least 30MB of RAM, since modern transformers create large dynamic activation layers which can’t be stored in flash or other read-only memory. Because Moonshine’s requirements scale with the size of the input window, we are on target to transcribe full sentences a few seconds long in 8MB of RAM or less.
I can’t wait to see what people are able to build with these new models, especially on resource-constrained platforms like the Raspberry Pi, where running full speech to text has been challenging. Please do get in touch if you’ve built something neat, we’d love to hear from you!
Update – I talk a bit more about Moonshine on YouTube at youtu.be/sZVTisKqJtA.