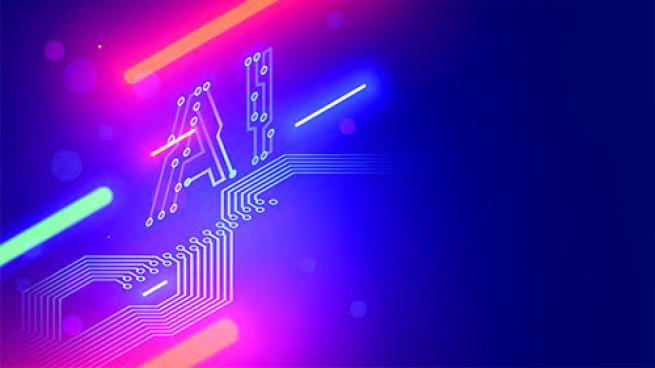
Embracing Private Cloud for Revenue Growth
One critical area where many travel and hospitality firms are falling short is in their adoption of private cloud solutions. While public cloud services are often perceived as an easier route to revenue generation due to their scalability and lower initial costs, private cloud environments offer unique advantages that can drive revenue growth.
Private clouds provide a level of customization and control that can lead to improved margins and more sustainable business expansion. By allowing companies to tailor their cloud infrastructure to meet specific needs, they can enhance competitive advantages and support strategic business goals more effectively. This flexibility is crucial for implementing AI solutions that often require secure and efficient handling of sensitive data.
Survey data reveals that 73% of IT decision-makers in the travel and hospitality sectors plan to invest between $500,000 and $5 million in AI this year. This represents a substantial increase in budgets compared to the previous year and highlights a growing recognition of AI’s potential to drive revenue. However, to fully realize this potential, companies should think holistically about how they can integrate AI with private cloud solutions.
Overcoming Barriers
Several barriers continue to hinder the effective implementation of AI across the travel and hospitality sector. Data management, cybersecurity, and trust in AI/ML outputs remain major concerns. 55% of survey respondents acknowledge the increasing importance of fraud detection and cybersecurity, while 52% express worries about data security risks associated with irresponsible AI practices. Data privacy is another significant worry, with 58% of respondents highlighting it as a key factor in responsible AI use.
Furthermore, 59% of IT leaders in the travel and hospitality industry identify cybersecurity as the greatest risk in AI adoption, while 68% believe that the accuracy of AI/ML systems needs substantial improvement before they can be fully trusted. These concerns underscore the need for a balanced approach that combines robust security measures with continuous improvement in AI accuracy and reliability.