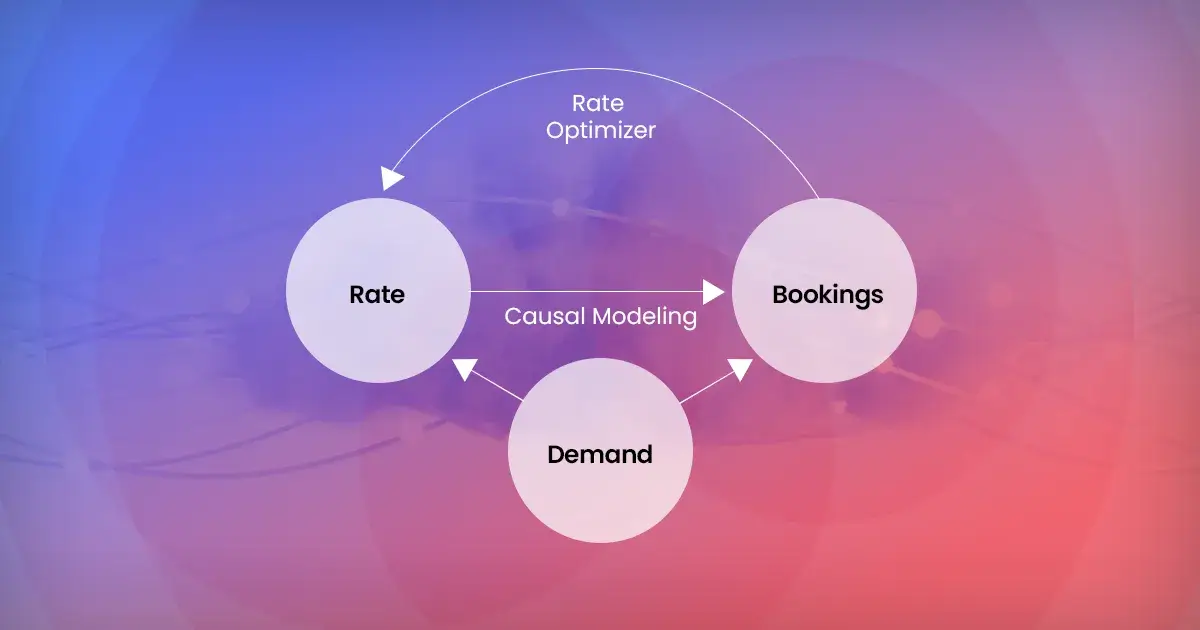
Imagine your city hosts a yearly festival, and you’re determining how to price your rooms for the upcoming year. Your revenue management system identifies that during the dates of the festival, there’s a spike in reservations and suggests that you increase room rates slightly during the festival period due to higher demand.
However, a deeper investigation incorporating real-time demand feeds reveals that undercutting the competition by 5% (while offering a special bundled package, including tickets and transportation to the festival) precisely influences bookings in a way that maximizes total revenue.
Why the opposing views? The initial suggestion uses a predictive machine learning algorithm designed to find statistical correlations in historical data. The result is a form of inductive reasoning: “When an outcome X happens, variable Y also happens. Therefore, Y likely causes X.”
However, correlation and causation are two different things, and confusing them can lead to misguided conclusions. These types of errors could be prevented with causal AI.
In this article, we explore how causal AI is being adopted across industries to help improve decision-making and its impact on hospitality.
What is causal AI?
Causal AI is a branch of artificial intelligence that uses distinct models and algorithms to uncover true cause-and-effect relationships. It analyzes billions of forward-looking data points to help guide real-time decision-making.
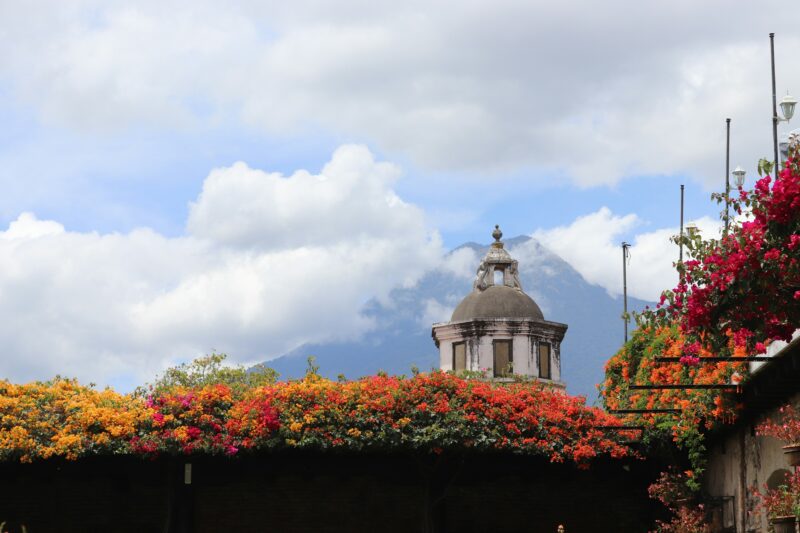
While correlation-based AI makes predictions by detecting patterns in historical data, causal AI understands why something happens, leading to more accurate insights.
How does causal AI work?
Causal AI is a sophisticated model that uses wider datasets and is designed to analyze more complex scenarios. For instance, in agriculture, a simple correlation between fertilizer use and crop yield wouldn’t be enough to make accurate predictions. Causal AI would incorporate external variables, such as soil quality and weather patterns, to provide a more comprehensive analysis.
The broader datasets are then processed using algorithms that identify cause-and-effect relationships and pinpoint the direct drivers of change. In the previous example, causal AI would account for the impact of soil quality to determine the necessary use of fertilizer to optimize crop yields. As well as providing insights, causal AI tells us the action required to achieve desired outcomes given current conditions.
Causal AI can also elaborate “what if” scenarios to predict outcomes from different combinations of variables. This is where it surpasses correlation-based AI, which is limited to historical data and struggles to predict novel scenarios caused by new patterns.
The key to causal AI is the data at hand. The more information the algorithm has to draw conclusions, the more accurate its predictions can be. In a world where we have more data than ever at our fingertips, it’s all about how to transform that data for real-world impact.
In summary, causal AI enhances decision-making thanks to its ability to:
a) Understand the real causes behind a current scenario
b) Predict future scenarios based on existing data and hypothetical factors
c) Reverse-engineer outcomes, determining the necessary conditions for a particular result, e.g., determining an optimal use of fertilizer to bring about a targeted crop yield.
How causal AI is being used across industries
Causal AI is an emerging technology that Gartner included among innovation triggers, which are still quite early in the hype cycle. It’s being successfully used in:
Healthcare
Causal AI is being used to identify the root causes of diseases and predict the effect of medication and lifestyle changes on patients. This allows for truly personalized treatments based on individual patient profiles. For example, the Jameel Clinic at MIT—uses causal AI, together with other machine learning technologies, to advance research in disease detection and drug discovery.
Finance
Causal AI helps financial institutions manage risks, anticipate economic downturns, and predict the effects of economic policies, such as the impact of interest rate changes on specific markets and overall inflation. Causality Link, for instance, analyzes thousands of documents in near real-time to identify cause-and-effect relationships.
Agriculture
In agriculture, causal AI is used to analyze causal relationships between farming practices—irrigation methods, fertilizer use, crop rotation, or pest control—and outcomes such as soil health, plant growth, and yields. It helps balance productivity and sustainability, making food production systems more resilient.
Manufacturing
Causal AI is used to identify the exact causes of inefficiencies or breakdowns by analyzing data from machinery, production lines, inventory levels, and shipping times. For example, BP not just predicts but also finds the root causes of component failure in wind turbines.
eCommerce
In eCommerce, causal modeling answers questions such as which groups are most price sensitive and can be tempted by a promotion or which ad visual should be served to which group. With causal AI, the biggest eCommerce players like Uber, Amazon, and Booking.com are optimizing multimillion-dollar marketing decisions.
Causal AI in hospitality
Causal AI is making strides across industries, and hospitality is no exception. While adoption has been slower, we’re starting to see the power behind causal AI emerge for hotels. For instance, it can help address complex questions that have traditionally been difficult to answer accurately, such as:
- How large a discount is needed to increase the likelihood of winning a particular customer back without unnecessarily cutting into revenue?
- How much do we need to undercut a competitor to increase bookings and maximize revenue rather than blindly matching the lowest rates?
- Why was revenue during the high season lower than expected? What would have happened if we had increased or decreased rates?
- What segments should we target with our upcoming marketing campaign, and what packages should we offer?
There are three areas of a hotel’s operations where causal AI is particularly promising.
1. Demand optimization
Demand optimization involves predicting future demand and capturing as much of it as possible at the right rate to maximize overall revenue. For their analysis, hotels typically use demand signals like seasonality, occupancy rates, and competitors’ prices. From there, they apply rule-based dynamic pricing, for example, trying to fill rooms at the highest possible rate when demand is high and discounting rates when demand is low.
The challenge with this approach is that it relies heavily on historical data, which may not always accurately reflect future scenarios, and tends to consider correlations in isolation (e.g., between rates and occupancy), which can lead to spurious conclusions.
With causal AI, it’s possible to integrate traditional demand signals and dynamic pricing with billions of forward-looking data points, such as local events and metasearch information, to detect popular search dates before a booking occurs.
Combined with a property’s historical booking patterns and guest demographics, causal AI can understand the causal link between rates, demand, and bookings and recommend a combination of actions, such as reducing rates or implementing marketing campaigns to get a close-to-perfect sell-out. This approach unifies the full range of pricing decision-making, including room rates, upsell opportunities, and promotions, both through OTA and direct bookings.
For example, if a hotel sees that competitors’ rates are trending down, causal AI might suggest launching a promotion to sell more rooms early in the booking window, minimizing revenue loss. Conversely, if competitors’ rates are trending up, the hotel can hold back rooms to sell later at higher rates, maximizing profit.
2. Marketing
Here are three hotel marketing areas where causal AI can make a difference:
Segmenting guests. Unlike traditional methods that segment guests by broad categories—such as business vs. leisure—, with causal AI, it’s possible to analyze patterns in guest data, such as demographics, booking behaviors, preferences, and even cart abandonment (when potential guests start but don’t complete a booking) to understand the driving factors that influence why certain guests book or abandon the process and take actions such as target those with highest travel intent for required dates.
Optimizing discounted rates. Causal AI analyzes how sensitive different guest segments are to price changes and determines the minimum discount needed to achieve the desired conversion rate. This allows hotels to attract price-sensitive guests without offering unnecessary discounts.
Evaluating campaign performance. Causal AI can analyze past marketing campaigns and guest data to identify the most effective channels, messages, upselling strategies, and timing to target guests and maximize conversion at the optimal price point.
3. Predictive maintenance
Smart sensors and IoT (Internet of Things) are not just for the manufacturing industry. Hotels, too, can use them to monitor the performance and usage patterns of HVAC units, boilers, and elevators, collecting data on pressure, temperature, usage patterns, and vibrations.
However, collecting data is only part of the equation; the real challenge is using it effectively. That’s where causal AI comes in. This technology can analyze the data to explain what caused past breakdowns, predict future failures, and recommend when to perform preventive maintenance before problems occur. The benefits include a longer lifespan of hotel equipment, improved guest experience, and lower energy costs.
Discover the power of Cloudbeds.